3.4. Newton Polynomial#
Assume that \(P_{n}(x)\) is the \(n\) degree polynomial such that
where \(x_{0}\), \(x_{1}\), \(x_{2}\), \ldots, \(x_{n}\) are distinct points. The \(P_{n}(x)\) can be expressed as follows,
or in Lagrange forms from the previous section.
Now, there are some challenges when it comes to using the above forms. For example, the coefficients in \(P_{n}(x)\) can be difficult to determine. As for Lagrange, if there is a new data entry the entire process needs to be recalculated. The use of nested multiplication and the relative ease of adding more data points for higher-order interpolating polynomials are advantages of Newton interpolation over Lagrange interpolating polynomials.
Newton polynomial can be also used to interpolate polynomials. This polynomial can be expressed as follows for a sets of distinct data points \(\{ x_{0}, x_{1},\ldots, x_{n}\}\).
where the coefficients \(a_{0}\), \(a_{1}\), \ldots, \(a_{n}\) can be indentured as follows. For example, to find \(a_{0}\),
Thus,
Also, for \(a_{1}\), we have,
The 0th Divided Difference of the function \(f\) with respect to \(x_{k}\) is defined as follows,
Similarly, the 1st Divided Difference of the function \(f\) with respect to \(x_{k}\) and \(x_{k+1}\) is defined as follows,
The 2nd Divided Difference of the function \(f\) with respect to \(x_{k}\), \(x_{k+1}\) and \(x_{k+2}\) is defined as follows,
The 3rd Divided Difference:
The jth Divided Difference:
The process ends with the single The nth Divided Difference:
Now, let \(a_{0} = f[x_{0}]\) and
to be Newton coefficients and Newton basis polynomials, respectively. Then, the Newton interpolation polynomial is a linear combination of Newton basis polynomials
The 1st and 2nd divided differences can be written in the form of a table.
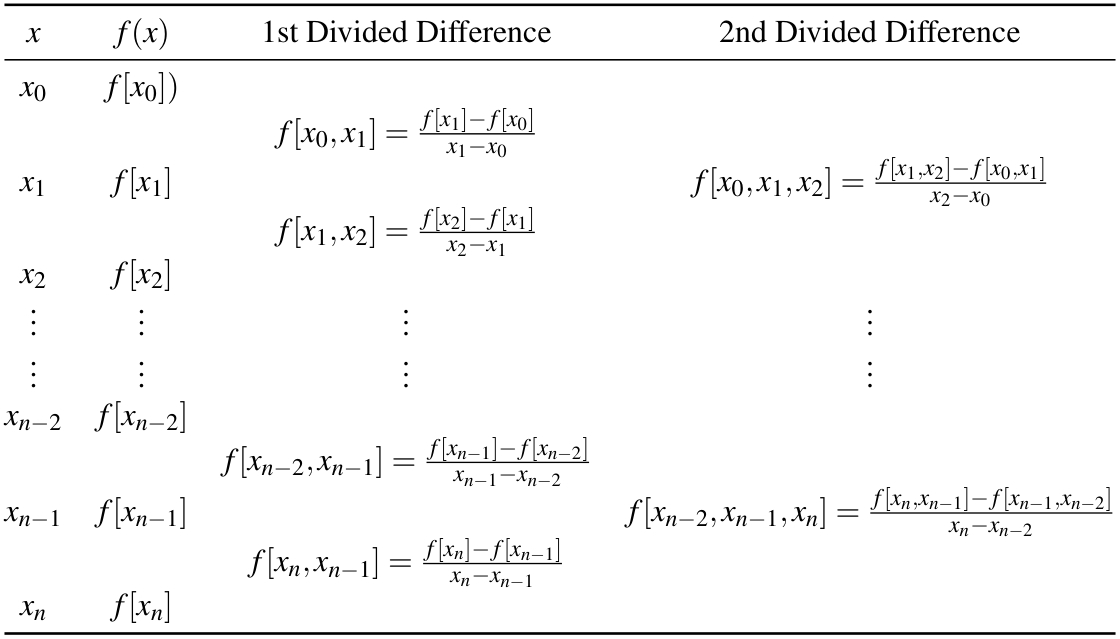
Fig. 3.2 Divided Differences for \(f(x)\)#
import numpy as np
import pandas as pd
def NewtonCoeff(xn, yn, Frame = False):
'''
Parameters
----------
xn : list/array
DESCRIPTION. a list/array consisting points x0, x1, x2,... ,xn
yn : list/array
DESCRIPTION. a list/array consisting points
y0 = f(x0), y1 = f(x1),... ,yn = f(xn)
Frame : TYPE, optional
DESCRIPTION. The default is False.
Returns
-------
TYPE frame or, coefs
DESCRIPTION.
'''
n = len(xn)
#Construct table and load xy pairs in first columns
A = np.zeros((n,n+1))
A[:,0]= xn[:]
A[:,1]= yn[:]
#Fill in Divided differences
for j in range(2,n+1):
for i in range(j-1,n):
A[i,j] = (A[i,j-1]-A[i-1,j-1]) / (A[i,0]-A[i-j+1,0])
#Copy diagonal elements into array for returning
a = np.zeros(n)
for k in range(0,n):
a[k] = A[k,k+1]
if Frame:
Cols = ['xi','yi']
temp = 'f[xi'
for i in range(A.shape[1]-2):
temp += ', xi+%i'% (i+1)
Cols.append(temp + ']')
Table = pd.DataFrame(A, columns=Cols)
return a, Table
else:
return a
def NewtonPoly(x, xn, yn):
'''
Parameters
----------
x : TYPE
DESCRIPTION.
xn : list/array
DESCRIPTION. a list/array consisting points x0, x1, x2,... ,xn
yn : list/array
DESCRIPTION. a list/array consisting points
y0 = f(x0), y1 = f(x1),... ,yn = f(xn)
Returns
-------
p : float
DESCRIPTION. P_n(x)
'''
n = len(xn)
# coefficients
a = NewtonCoeff(xn, yn)
p = a[n-1]
for i in range(n-2,-1,-1):
p = p*(x-xn[i]) + a[i]
return p
function [a] = NewtonCoeff(xn, yn)
%{
Parameters
----------
xn : list/array
DESCRIPTION. a list/array consisting points x0, x1, x2,... ,xn
yn : list/array
DESCRIPTION. a list/array consisting points
y0 = f(x0), y1 = f(x1),... ,yn = f(xn)
Frame : TYPE, optional
DESCRIPTION. The default is False.
Returns
-------
TYPE a list
DESCRIPTION. coefs
%}
n = length(xn);
% Construct table and load xy pairs in first columns
A = zeros(n,n);
A(:,1)= yn;
% Fill in Divided differences
for i = 2:n
for j = 2:i
A(i,j)=(A(i,j-1)-A(i-1,j-1))/(xn(i)-xn(i-j+1));
end
end
a = diag(A);
end
function p = NewtonPoly(x, xn, yn)
%{
Parameters
----------
x : TYPE
DESCRIPTION.
xn : list/array
DESCRIPTION. a list/array consisting points x0, x1, x2,... ,xn
yn : list/array
DESCRIPTION. a list/array consisting points
y0 = f(x0), y1 = f(x1),... ,yn = f(xn)
Returns
-------
p : float
DESCRIPTION. P_n(x)
Example:
xn = [-2, 1 , 3 , 5 , 6, 7];
yn = [-5, -3 ,-1 , 1 , 4, 10]
x = linspace(min(xn)-1 , max(xn)+1 , 100);
%}
n = length(xn);
% coefficients
a = NewtonCoeff(xn, yn);
p = a(n);
for i = n-1:-1:1
p = p.*(x-xn(i)) + a(i);
end
end
Example: Consider the following data points
Construct an interpolating polynomial using the Newton divided-difference formula and all of this data.
Solution:
# This part is used for producing tables and figures
import sys
sys.path.insert(0,'..')
import hd_tools as hd
import numpy as np
from hd_Interpolation_Algorithms import NewtonPoly, NewtonCoeff
# A set of distinct points
xn = np.array ([-2, 1 , 3 , 5 , 6, 7])
yn = np.array ([-5, -3 ,-1 , 1 , 4, 10])
_, Table = NewtonCoeff(xn, yn, Frame = True)
display(Table.style.set_properties(subset=['xi', 'yi'], **{'background-color': 'black', 'color': 'PaleGreen',
'border-color': 'DarkGreen'}).format(dict(zip(Table.columns.tolist(),len(Table.columns)*["{:.4f}"]))))
x = np.linspace( xn.min()-1 , xn.max()+1 ,100)
y = NewtonPoly( x , xn , yn )
hd.interpolation_method_plot(xn, yn, x, y, title = 'Newton Method')
xi | yi | f[xi, xi+1] | f[xi, xi+1, xi+2] | f[xi, xi+1, xi+2, xi+3] | f[xi, xi+1, xi+2, xi+3, xi+4] | f[xi, xi+1, xi+2, xi+3, xi+4, xi+5] | |
---|---|---|---|---|---|---|---|
0 | -2.0000 | -5.0000 | 0.0000 | 0.0000 | 0.0000 | 0.0000 | 0.0000 |
1 | 1.0000 | -3.0000 | 0.6667 | 0.0000 | 0.0000 | 0.0000 | 0.0000 |
2 | 3.0000 | -1.0000 | 1.0000 | 0.0667 | 0.0000 | 0.0000 | 0.0000 |
3 | 5.0000 | 1.0000 | 1.0000 | 0.0000 | -0.0095 | 0.0000 | 0.0000 |
4 | 6.0000 | 4.0000 | 3.0000 | 0.6667 | 0.1333 | 0.0179 | 0.0000 |
5 | 7.0000 | 10.0000 | 6.0000 | 1.5000 | 0.2083 | 0.0125 | -0.0006 |
Theorem
Assume that \(f \in C^n[a, b]\) and data points \(\{ x_{0}, x_{1},\ldots, x_{n}\}\) from \([a,b]\) are distinct. Then, there is a number \(\xi \in (a, b)\) such that
Proof:
Define \(g(x) = f(x) - P_{n}(x)\). Now, since \(f(x) = P_{n}(x)\) for all \(x \in \{ x_{0}, x_{1},\ldots, x_{n}\}\), then \(g(x) = 0\) for all \(x \in \{ x_{0}, x_{1},\ldots, x_{n}\}\). Therefore, \(g(x)\) has \(n+1\) unique zeros in \([a,b]\). It follows from Generalized Rolle’s Theorem that there is a number \(\xi \in (a, b)\) such that
On the other hand, Since \(P_{n}(x)\) is a \(n\) degree polynomial with leading coefficient is \(f[x_{0},x_{1},\ldots ,~x_{{n}}]\). Therefore,
Thus,
3.4.1. Newton Forward Differences#
Recall that forward difference notation \(\Delta\). Given equidistantly distributed data points, we can define Newton forward differences. Note that this is a particular case of Newton polynomials. Given n data points, we have,
where \(y_{j} = f(x_{j})\) for \(0\leq j \leq n\) and
Note that \(h = \Delta x_{j} = x_{j+1} -x_{j}\). Then, the divided differences can be calculated via forward differences defined as
Therefore, the Newton Forward-Difference Formula can be found as follows,
where \(s = \dfrac{x - x_{0}}{h}\). The above equation can be also expressed as follows,
import numpy as np
import pandas as pd
from scipy.special import binom
def ForwardNewton(x, xn, yn, Frame = False):
'''
Parameters
----------
x : float
DESCRIPTION. point x
xn : list/array
DESCRIPTION. a list/array consisting points x0, x1, x2,... ,xn
yn : list/array
DESCRIPTION. a list/array consisting points
y0 = f(x0), y1 = f(x1),... ,yn = f(xn)
Frame : TYPE, optional
DESCRIPTION. The default is False.
Returns
-------
TYPE frame or, coefs
DESCRIPTION.
'''
h = xn[1]- xn[0]
n = xn.shape[0]
A = np.zeros([n,n],dtype = float)
A[:,0] = yn
for i in range(1, n):
A[i:,i] = (A[i:,i-1] - A[i-1:-1,i-1])
df = np.diag(A)
# Newton's forward difference formula for variable x
s = (x - xn[0])/h
Pn = df[0]
for i in range(1, len(df)):
Pn += binom(s, i)*df[i]
if Frame:
Cols = ['xi','yi']
for i in range(A.shape[1]-1):
Cols.append('Delta^%i fi'% (i+1))
Table = pd.DataFrame(np.insert(A, 0, xn, axis=1), columns=Cols)
return Pn, Table
else:
return Pn
function [Pn] = ForwardNewton(x, xn, yn)
%{
Parameters
----------
xn : list/array
DESCRIPTION. a list/array consisting points x0, x1, x2,... ,xn
yn : list/array
DESCRIPTION. a list/array consisting points
y0 = f(x0), y1 = f(x1),... ,yn = f(xn)
Frame : TYPE, optional
DESCRIPTION. The default is False.
Returns
-------
TYPE frame or, coefs
DESCRIPTION.
xn = [-1, 1 , 3 , 5 , 7, 9];
yn = [ -15, -3, -47, -99, -111, -35];
x = linspace(min(xn)-1 , max(xn)+1 , 100);
%}
h = xn(2)- xn(1);
n = length(xn);
A = zeros([n,n]);
A(:, 1) = yn;
for i =2:n
A(i:n,i) = (A(i:n,i-1) - A((i-1):(n-1),i-1));
end
df = diag(A);
% Newton's forward difference formula for variable x
s = (x - xn(1))/h;
Pn = df(1);
for k = 2:length(df)
Pn = Pn + newforward_term (s, k, df);
end
function [out] = newforward_term (s, k, df)
% Newton's forward difference formula coeff
if k == 1
out = df(k);
elseif k ==2
out = s.*df(k)/ factorial(k-1);
else
c = s;
for j=1:(k-2)
c = c.* (s-j);
end
out = (c.*df(k))/ factorial(k-1);
end
Example: Consider the following data points
Construct an interpolating polynomial using the Newton forward-difference formula and all of this data.
import numpy as np
from hd_Interpolation_Algorithms import ForwardNewton
# A set of distinct points
xn = np.array ([-1, 1 , 3 , 5 , 7, 9])
yn = np.array([-15, -3, -47, -99, -111, -35])
x = np.linspace( xn.min()-1 , xn.max()+1 ,100)
y, Table = ForwardNewton(x, xn, yn, Frame = True)
display(Table.style.set_properties(subset=['xi', 'yi'], **{'background-color': 'black', 'color': 'PaleGreen',
'border-color': 'DarkGreen'}).format(dict(zip(Table.columns.tolist(),len(Table.columns)*["{:.4f}"]))))
hd.interpolation_method_plot(xn, yn, x, y, title = 'Forward Newton Method')
xi | yi | Delta^1 fi | Delta^2 fi | Delta^3 fi | Delta^4 fi | Delta^5 fi | |
---|---|---|---|---|---|---|---|
0 | -1.0000 | -15.0000 | 0.0000 | 0.0000 | 0.0000 | 0.0000 | 0.0000 |
1 | 1.0000 | -3.0000 | 12.0000 | 0.0000 | 0.0000 | 0.0000 | 0.0000 |
2 | 3.0000 | -47.0000 | -44.0000 | -56.0000 | 0.0000 | 0.0000 | 0.0000 |
3 | 5.0000 | -99.0000 | -52.0000 | -8.0000 | 48.0000 | 0.0000 | 0.0000 |
4 | 7.0000 | -111.0000 | -12.0000 | 40.0000 | 48.0000 | 0.0000 | 0.0000 |
5 | 9.0000 | -35.0000 | 76.0000 | 88.0000 | 48.0000 | 0.0000 | 0.0000 |
Now, using the table, we have,
3.4.2. Newton Backward Differences#
Similarly, assume that data points are equidistant.
where \(y_{j} = f(x_{j})\) for \(0\leq j \leq n\) and
Recall that \(h = \nabla x_{j} = x_{j} -x_{j-1}\). The backward difference formula can be defined as follows,
Therefore, the Newton Backward-Difference Formula can be found as follows,
Now, assume that we can extend the binomial coefficient to include all real numbers,
Then,
where \(s = \dfrac{x - x_{n}}{h}\).
def BackwardNewton(xn, yn, table = False):
'''
Input: data points
Output: Newton coefficients
'''
h = xn[1]- xn[0]
n = xn.shape[0]
A = np.zeros([n,n],dtype = float)
A[:,0] = yn
B = A.copy()
for i in range(1, n):
A[i:,i] = (A[i:,i-1] - A[i-1:-1,i-1])
B[:-i,i] = (A[i:,i-1] - A[i-1:-1,i-1])
df = A[-1,:]
A = B.copy()
del B
# Newton's forward difference formula for variable x
def Pn(x0, df = df, h = h, xn = xn):
s = (x0 - xn[-1])/h
P = df[0]
for i in range(1, len(df)):
if df[i]!=0:
T = s
for j in range(1, i):
T *= (s+j)
T /= np.math.factorial(i)
P += T*df[i]
del T
return P
if table:
Cols = ['xi','yi']
for i in range(A.shape[1]-1):
Cols.append('Delta^%i fi'% (i+1))
Table = pd.DataFrame(np.insert(A, 0, xn, axis=1), columns=Cols)
return Pn, Table
else:
return Pn
import numpy as np
import pandas as pd
from scipy.special import binom
def BackwardNewton(x, xn, yn, Frame = False):
'''
Parameters
----------
x : float
DESCRIPTION. point x
xn : list/array
DESCRIPTION. a list/array consisting points x0, x1, x2,... ,xn
yn : list/array
DESCRIPTION. a list/array consisting points
y0 = f(x0), y1 = f(x1),... ,yn = f(xn)
Frame : TYPE, optional
DESCRIPTION. The default is False.
Returns
-------
TYPE frame or, coefs
DESCRIPTION.
'''
h = xn[-1] - xn[-2]
n = xn.shape[0]
A = np.zeros([n,n],dtype = float)
A[:,0] = yn
for i in range(1, n):
A[i:,i] = (A[i:,i-1] - A[i-1:-1,i-1])
df = A[-1,:]
# Newton's forward difference formula for variable x
s = (x - xn[-1])/h
Pn = df[0]
for i in range(1, len(df)):
Pn += binom(-s, i)*df[i]*(-1)**(i)
if Frame:
Cols = ['xi','yi']
for i in range(A.shape[1]-1):
Cols.append('Delta^%i fi'% (i+1))
Table = pd.DataFrame(np.insert(A, 0, xn, axis=1), columns=Cols)
return Pn, Table
else:
return Pn
function [Pn] = BackwardNewton(x, xn, yn)
%{
Parameters
----------
xn : list/array
DESCRIPTION. a list/array consisting points x0, x1, x2,... ,xn
yn : list/array
DESCRIPTION. a list/array consisting points
y0 = f(x0), y1 = f(x1),... ,yn = f(xn)
Frame : TYPE, optional
DESCRIPTION. The default is False.
Returns
-------
TYPE frame or, coefs
DESCRIPTION.
xn = [-1, 1 , 3 , 5 , 7, 9];
yn = [ -15, -3, -47, -99, -111, -35];
x = linspace(min(xn)-1 , max(xn)+1 , 100);
%}
h = xn(end) - xn(end-1);
n = length(xn);
A = zeros([n,n]);
A(:, 1) = yn;
for i =2:n
A(i:n,i) = (A(i:n,i-1) - A((i-1):(n-1),i-1));
end
df = A(end,:);
% Newton's forward difference formula for variable x
s = (x - xn(end))/h;
Pn = df(1);
for k = 2:length(df)
Pn = Pn + newbackward_term (s, k, df);
end
function [out] = newbackward_term (s, k, df)
% Newton's forward difference formula coeff
if k == 1
out = df(k);
elseif k ==2
out = s.*df(k)/ factorial(k-1);
else
c = s;
for j=1:(k-2)
c = c.* (s+j);
end
out = ((c.*df(k))/ factorial(k-1));
end
Example: Consider the following data points
Construct an interpolating polynomial using Backward Newton’s differences that uses all this data.
import numpy as np
from hd_Interpolation_Algorithms import BackwardNewton
# A set of distinct points
xn = np.array ([-1, 1 , 3 , 5 , 7, 9])
yn = np.array([-15, -3, -47, -99, -111, -35])
x = np.linspace( xn.min()-1 , xn.max()+1 ,100)
y, Table = BackwardNewton(x, xn, yn, Frame = True)
display(Table.style.set_properties(subset=['xi', 'yi'], **{'background-color': 'black', 'color': 'PaleGreen',
'border-color': 'DarkGreen'}).format(dict(zip(Table.columns.tolist(),len(Table.columns)*["{:.4f}"]))))
hd.interpolation_method_plot(xn, yn, x, y, title = 'Backward Newton Method')
xi | yi | Delta^1 fi | Delta^2 fi | Delta^3 fi | Delta^4 fi | Delta^5 fi | |
---|---|---|---|---|---|---|---|
0 | -1.0000 | -15.0000 | 0.0000 | 0.0000 | 0.0000 | 0.0000 | 0.0000 |
1 | 1.0000 | -3.0000 | 12.0000 | 0.0000 | 0.0000 | 0.0000 | 0.0000 |
2 | 3.0000 | -47.0000 | -44.0000 | -56.0000 | 0.0000 | 0.0000 | 0.0000 |
3 | 5.0000 | -99.0000 | -52.0000 | -8.0000 | 48.0000 | 0.0000 | 0.0000 |
4 | 7.0000 | -111.0000 | -12.0000 | 40.0000 | 48.0000 | 0.0000 | 0.0000 |
5 | 9.0000 | -35.0000 | 76.0000 | 88.0000 | 48.0000 | 0.0000 | 0.0000 |
Using the table, we have,