3.3. Interpolation and Lagrange polynomial#
3.3.1. Lagrange Interpolating Polynomials#
For a distinct set of \(n + 1\) data points (no two \(x_{j}\) are the same)
the Lagrange basis polynomials are defined as follows,
where \(y_{j} = f(x_{j})\) and \(0\leq j\leq n\).
Furthermore, the interpolation polynomial in the Lagrange form is a linear combination of these Lagrange basis polynomials
Theorem
Assuming that \(x_0\), \(x_1\), … , \(x_{n}\) are \(n+1\) distinct numbers from \([a, b]\) and \(f \in C^{n+1} [a,b]\). Then, there exists a number \(\xi(x_{j}) \in (x_{0},x_{1})\bigcup \ldots \bigcup (x_{n-1},x_{n})\) such that
where \(L(x)\) is the interpolating polynomial provided in equation (3.16).
Proof:
Note that \(f(x_{j}) = L(x_{j})\) when \(0\leq j \leq n\). Therefore, when \(x = x_{j}\) when \(0\leq j \leq n\) for any \(\xi(x_{j}) \in (x_{0},x_{1})\bigcup \ldots \bigcup (x_{n-1},x_{n})\) yields equation (3.17).
Now, when \(x \neq x_{j}\) for \(j=0,1,\ldots, n\), define a function \(g(t)\) for \(t\in[a,b]\) as follows,
Since \(f \in C^{n+1}[a, b]\), and \(P \in C^{\infty}[a, b]\), then \(g \in C^{n+1}[a, b]\). Now, for \(t = x_{k}\) for \(k=0,1,\ldots, n\), we have
Moreover, when \(t = x\), we have,
Therefore, \(g \in C^{n+1}[a, b]\) and \(g\) is zero at \(n+2\) distinct points \(x\), \(x_0\), \(x_1\), … , \(x_n\). By Generalized Rolle’s Theorem, there is a number \(\xi\in (a, b)\) such that
It follows that
On the other hand, \(\prod _{j = 0 }^{n}\frac{(t - x_j)}{(x - x_j)}\) is a \(n+1\) degree polynomial and its first \((n+1)\) are equal to zero. We have,
The derivative of those lower-degree terms would be zero, and
Solving the above equation for \(f(x\), we have,
Corollary
In addition to assumptions provided in Theorem \ref{Lagrange_Error_theorem}, assume that
for some values of \(M\) and all points \(\xi(x) \in (x_{0},~x_{1})\bigcup \ldots \bigcup (x_{n-1},~x_{n})\), then
import numpy as np
def LagrangePolyCoeff (x ,i , xn):
'''
Parameters
----------
x : float
DESCRIPTION. point x
i : int
DESCRIPTION. index of L_{i}
xn : list/array
DESCRIPTION. a list/array consisting points x0, x1, x2,... ,xn
Returns
-------
Lj : TYPE
DESCRIPTION.
'''
Lj=1
for j in range (len(xn)):
if i!=j:
Lj*=( x-xn[j])/( xn[i]-xn[j])
return Lj
def LagrangePoly(x , xn , yn ):
'''
Parameters
----------
x : float
DESCRIPTION. point x
xn : list/array
DESCRIPTION. a list/array consisting points x0, x1, x2,... ,xn
yn : list/array
DESCRIPTION. a list/array consisting points
y0 = f(x0), y1 = f(x1),... ,yn = f(xn)
Returns
-------
L : float
DESCRIPTION. L(x)
'''
LagrangePoly = np.array ([LagrangePolyCoeff(x ,i , xn ) for i in range (len(xn))])
L = np.dot( yn , LagrangePoly)
return L
function [Lj] = LagrangePolyCoeff(x ,i , xn)
%{
Parameters
----------
x : float
DESCRIPTION. point x
i : int
DESCRIPTION. index of L_{i}
xn : list/array
DESCRIPTION. a list/array consisting points x0, x1, x2,... ,xn
Returns
-------
Lj : TYPE
DESCRIPTION.
%}
Lj=1;
for j =1:length(xn)
if i ~= j
Lj = Lj .*( x - xn(j))/( xn(i) - xn(j));
end
end
function [L] = LagrangePoly(x , xn , yn)
%{
Parameters
----------
x : float
DESCRIPTION. point x
xn : list/array
DESCRIPTION. a list/array consisting points x0, x1, x2,... ,xn
yn : list/array
DESCRIPTION. a list/array consisting points
y0 = f(x0), y1 = f(x1),... ,yn = f(xn)
Returns
-------
L : float
DESCRIPTION. L(x)
Example:
xn = [1 ,2 ,3 ,4 ,5 , 6];
yn = [-3 ,0 ,-1 ,2 ,1 , 4];
x = linspace(min(xn)-1 , max(xn)+1 , 100);
%}
LagrangePoly = zeros(length(xn), length(x));
for i =1:length(xn)
LagrangePoly(i,:) = LagrangePolyCoeff(x, i , xn );
end
L = 0.*x;
for j =1:length(x)
L(j) = dot(yn, LagrangePoly(:,j));
end
Example: Consider the following data points
and construct an interpolating polynomial using Lagrange polynomial and all of this data.
Solution:
Calculating \(L_{j}\)s with \(n = 3\) for \(j=0,1,2,3\):
Similarly, we can calculate all \(L_{j}\)s:
Moreover,
# This part is used for producing tables and figures
import sys
sys.path.insert(0,'..')
import hd_tools as hd
import numpy as np
from hd_Interpolation_Algorithms import LagrangePoly
# A set of distinct points
xn = np.array ([1 ,2 ,3 ,4 ,5 , 6])
yn = np.array ([-3 ,0 ,-1 ,2 ,1 , 4])
x = np.linspace(xn.min()-1 , xn.max()+1 , 100)
y = LagrangePoly(x , xn , yn )
# Plots
hd.interpolation_method_plot(xn, yn, x, y, title = 'Lagrange Interpolating Polynomials')
Example: Assume that \(x_{0}<x_{1}\) are distinct numbers in \([a,~b]\) and \(f \in C^{2} [a,~b]\). Also, assume that Lagrange the interpolation \(L(x)\) only comprises two data points \(\{(x_{0},y_{0}),~(x_{1},y_{1})\}\). Then, demonstrate the following using the error formula for the Lagrange interpolation:
where \( \displaystyle{M = \max_{x_0\leq x\leq x_1}} |f''(x)|\) and \(h = x_1 - x_0\).
Solution: The Lagrange interpolating polynomial using two data points \(\{(x_{0},y_{0}),~(x_{1},y_{1})\}\):
The error formula \eqref{Lagrange_Error_theorem} is updated by utilizing the provided data point.
where \(\xi(x)\) is a unknown point between \(x_{0}\) and \(x_{1}\). Hence
Because \(\xi(x)\) is unknown, the value of \(f''(\xi(x))\) cannot be calculated precisely. We may, however, limit the error by finding the largest possible value for \(|f''(\xi(x))|\). Let
Then, the bound of \(|f''(x)|\) on \([x_{0},~x_{1}] \) can be obtained as follows,
Therefore,
Observe that the maximum of the function \(g(x) = (x - x_{0})(x - x_{1})\) in \([x_{0},~x_{1}]\) appears at the critical point \(x = \dfrac{(x_{0} + x_{1})}{2}\) as
and it follows from setting \(g'(x) = 0\) that
Thus the maximum of \(g\) on \((x_{0},~x_{1})\) is \(|(x - x_{0})(x - x_{1})| = (x_{1} - x_{0})^2/4\). Therefore,
As a result, for each \(x \in [x_{0},~x_{1}]\), we obtain
Now, since \(h = x_1 - x_0\),
Example: Consider the following table having the data for \(f(x) = e^{x} + \sin(2x)\) on \([0.1,~0.5]\):
\(x_i\) |
0.1000 |
0.3000 |
0.5000 |
---|---|---|---|
\(y_i\) |
1.3038 |
1.9145 |
2.4902 |
a. Use Lagrange polynomials to interpolate the points.
b. Find the approximation of \(f(0.4)\) using the Lagrange interpolated polynomial and estimate an error bound and absolute error for the approximation.
Solution:
\begin{enumerate}[label=\alph*), labelindent=0pt] a. Calculating \(L_{j}\)s with \(n = 2\) for \(j=0,1,2\):
Observe that in the above computations, we multiplied both numerator and denominator by 10 for convenience. Moreover,
b. First,
while \(f(0.4) = 2.209181\). Therefore, the absolute error here is
Now to compute an error bound of the approximation, we use the following formula
Taking the third derivative of the given function, we get
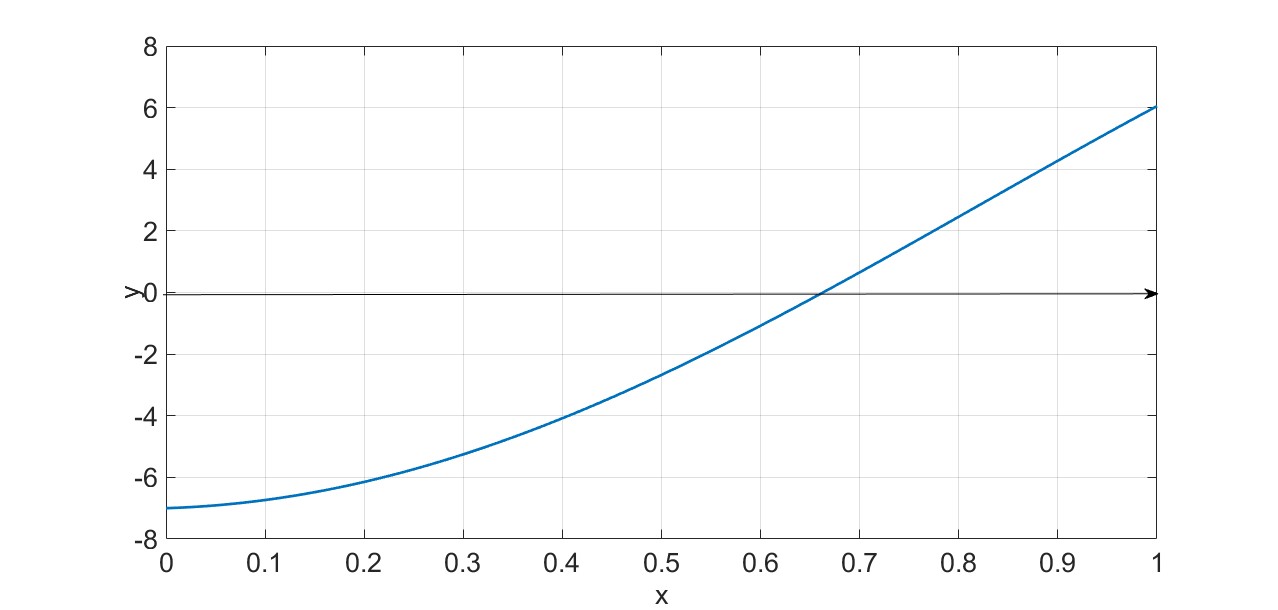
As can be seen \(f'''(x)\) is an increasing function when \(x \in (0.1, 0.5)\). Thus
takes its maximum at \(x = 0.1\), and
Therefore, the error bound at \(x = 0.4\):
As can be seen, the maximum estimate error is larger than the actual absolute error at \(0.4\) as the error bound needs to highlight the maximum expected error!