5.2. DataFrame and Series Indices#
5.2.1. DataFrame Indices#
In Pandas, a DataFrame is a two-dimensional labeled data structure with columns that can be of different data types. Each column in a DataFrame is a Pandas Series, and the entire DataFrame has both row and column indices [Pandas Developers, 2023].
Row indices can be customized or left as default. The default row index is a sequence of integers starting from 0. However, you can set a specific column to be the index or assign custom index labels to rows.
Example:
import pandas as pd
import numpy as np
import string
# Define the number of rows
n = 10
# Generate random data with n rows and 2 columns
data = np.random.randint(0, 100, size=(n, 2))
# Generate letters from A to Z for index labels
index_labels = list(string.ascii_uppercase)[:n]
# Create a DataFrame with random data, named columns, and custom index
df = pd.DataFrame(data = data,
columns=['Col 1', 'Col 2'],
index=index_labels)
# Print the DataFrame
print("Generated DataFrame:")
display(df)
Generated DataFrame:
Col 1 | Col 2 | |
---|---|---|
A | 95 | 22 |
B | 26 | 70 |
C | 68 | 35 |
D | 22 | 17 |
E | 92 | 69 |
F | 35 | 14 |
G | 74 | 31 |
H | 71 | 94 |
I | 39 | 20 |
J | 25 | 73 |
5.2.2. Series Indices#
A Series is a one-dimensional labeled array in Pandas. Like DataFrames, Series also have indices, which provide labels for each element in the Series. The default index for a Series is similar to the row index in a DataFrame (a sequence of integers starting from 0). However, you can customize the index with labels [Pandas Developers, 2023].
Example:
import pandas as pd
import numpy as np
import string
# Define the number of rows
n = 10
# Generate random data with n rows
data = np.random.randint(0, 100, size=n)
# Generate letters from A to Z for index labels
index_labels = list(string.ascii_uppercase)[:n]
# Create a Pandas Series with random data and custom index
series = pd.Series(data, index=index_labels)
# Print the Series
display(series)
A 17
B 74
C 37
D 85
E 6
F 57
G 44
H 34
I 26
J 85
dtype: int32
Indices are crucial in Pandas as they enable powerful data alignment during operations. When performing operations on DataFrames or Series, Pandas uses the indices to match elements correctly, even if the data is not in the same order.
Indices can be used for selection, alignment, merging, and other operations, making data manipulation more intuitive and accurate in Pandas.
5.2.3. Index Alignment in Pandas#
Index alignment is a powerful feature in Pandas that facilitates seamless and efficient data manipulation and computation across Series and DataFrames. When performing operations involving multiple data structures, Pandas aligns the data based on their indices, ensuring that calculations occur between corresponding elements [Pandas Developers, 2023].
This alignment is crucial for accurately combining, comparing, and performing arithmetic operations on data with different structures but related indices.
Example - Series Alignment:
import pandas as pd
# Create two Pandas Series
data1 = pd.Series([10, 20, 30], index=['A', 'B', 'C'])
data2 = pd.Series([5, 15, 25], index=['B', 'C', 'D'])
# Perform element-wise addition on the Series
result = data1 + data2
# Display the result using the appropriate function for a Series
print(result)
A NaN
B 25.0
C 45.0
D NaN
dtype: float64
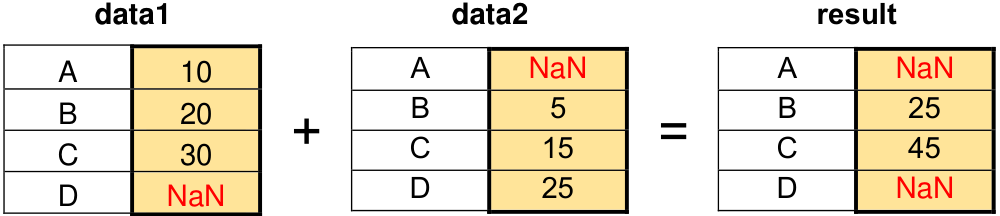
Fig. 5.1 Index Alignment Example.#
In this example, the Series data1
and data2
have different indices. However, when the addition operation is performed, Pandas aligns the data based on their indices. As a result, calculations are only performed where indices match, and NaN (Not a Number) values are introduced for indices that do not match.
In data analysis, “NaN” stands for “Not a Number.” It is a special value used to represent missing or undefined data in numerical or floating-point data types.
5.2.4. Various Types of Indices#
5.2.4.1. Numeric Index#
A Numeric Index holds all NumPy numeric dtypes except float16
. It is primarily used for indexing and aligning numeric data [Pandas Developers, 2023].
Example:
import pandas as pd
# Creating a DataFrame with a Numeric Index
numeric_index = pd.Index([1.1, 2.2, 3.3, 4.4, 5.5])
df_numeric = pd.DataFrame({'Values': [10, 20, 30, 40, 50]}, index=numeric_index)
display(df_numeric)
Values | |
---|---|
1.1 | 10 |
2.2 | 20 |
3.3 | 30 |
4.4 | 40 |
5.5 | 50 |
5.2.4.2. CategoricalIndex#
A CategoricalIndex is based on an underlying Categorical
type. It can take on a limited, fixed number of possible values (categories). It might have an inherent order, but numerical operations are not supported [Pandas Developers, 2023].
Example:
import pandas as pd
# Creating a DataFrame with a CategoricalIndex
categorical_index = pd.CategoricalIndex(['a', 'b', 'c', 'a', 'b', 'c'])
df_categorical = pd.DataFrame({'Values': [10, 20, 30, 40, 50, 60]}, index=categorical_index)
display(df_categorical)
Values | |
---|---|
a | 10 |
b | 20 |
c | 30 |
a | 40 |
b | 50 |
c | 60 |
5.2.4.3. IntervalIndex#
An IntervalIndex is an immutable index of intervals that are closed on the same side. It is used to represent intervals of time or other continuous data [Pandas Developers, 2023].
Example:
import pandas as pd
# Creating a DataFrame with an IntervalIndex
interval_index = pd.interval_range(start=0, end=5)
df_interval = pd.DataFrame({'Values': [10, 20, 30, 40, 50]}, index=interval_index)
display(df_interval)
Values | |
---|---|
(0, 1] | 10 |
(1, 2] | 20 |
(2, 3] | 30 |
(3, 4] | 40 |
(4, 5] | 50 |
5.2.4.4. MultiIndex#
A MultiIndex is a multi-level, or hierarchical, index object that allows higher-dimensional data to be represented in a lower-dimensional DataFrame structure [Pandas Developers, 2023].
Example:
import pandas as pd
# Creating a DataFrame with a MultiIndex
arrays = [['bar', 'bar', 'baz', 'baz', 'foo', 'foo', 'qux', 'qux'],
['one', 'two', 'one', 'two', 'one', 'two', 'one', 'two']]
tuples = list(zip(*arrays))
multi_index = pd.MultiIndex.from_tuples(tuples, names=['first', 'second'])
df_multi = pd.DataFrame({'Values': [10, 20, 30, 40, 50, 60, 70, 80]}, index=multi_index)
display(df_multi)
Values | ||
---|---|---|
first | second | |
bar | one | 10 |
two | 20 | |
baz | one | 30 |
two | 40 | |
foo | one | 50 |
two | 60 | |
qux | one | 70 |
two | 80 |
5.2.4.5. DatetimeIndex#
A DatetimeIndex is an immutable array-like structure of datetime64
data. It is used for indexing and aligning datetime data [Pandas Developers, 2023].
Example:
import pandas as pd
# Creating a DataFrame with a DatetimeIndex
datetime_index = pd.DatetimeIndex(['2020-01-01 10:00:00', '2020-02-01 11:00:00'])
df_datetime = pd.DataFrame({'Values': [10, 20]}, index=datetime_index)
display(df_datetime)
Values | |
---|---|
2020-01-01 10:00:00 | 10 |
2020-02-01 11:00:00 | 20 |
5.2.4.6. TimedeltaIndex#
A TimedeltaIndex is an immutable index of timedelta64
data, which represents differences in time. It is used for indexing and aligning time durations [Pandas Developers, 2023].
Example:
import pandas as pd
# Creating a DataFrame with a TimedeltaIndex
timedelta_index = pd.TimedeltaIndex(['0 days', '1 days', '2 days', '3 days', '4 days'])
df_timedelta = pd.DataFrame({'Values': [10, 20, 30, 40, 50]}, index=timedelta_index)
display(df_timedelta)
Values | |
---|---|
0 days | 10 |
1 days | 20 |
2 days | 30 |
3 days | 40 |
4 days | 50 |
5.2.4.7. PeriodIndex#
A PeriodIndex is an immutable array that holds ordinal values indicating regular periods of time. Each index key is boxed to a Period
object, which carries metadata such as frequency information [Pandas Developers, 2023].
Example:
import pandas as pd
# Creating a DataFrame with a PeriodIndex
period_index = pd.PeriodIndex.from_fields(year=[2000, 2002], quarter=[1, 3])
df_period = pd.DataFrame({'Values': [10, 20]}, index=period_index)
display(df_period)
Values | |
---|---|
2000Q1 | 10 |
2002Q3 | 20 |
Table 5.3 provides a concise summary of each index type along with a brief description [Pandas Developers, 2023].
Index Type |
Description |
---|---|
Numeric Index |
Holds all NumPy numeric dtypes except |
CategoricalIndex |
Based on an underlying |
IntervalIndex |
Immutable index of intervals closed on the same side, represents intervals of time or continuous data. |
MultiIndex |
Multi-level, hierarchical index object, allows higher-dimensional data in lower-dimensional DataFrame structure. |
DatetimeIndex |
Immutable array-like structure of |
TimedeltaIndex |
Immutable index of |
PeriodIndex |
Immutable array holding ordinal values indicating regular periods in time, each key is boxed to a |